How is AI Impacting drug discovery?
We spoke to Colin Brown(CB), Chief Scientific Officer; Valeria Chichagova (VC), Director of Technology and Mike Nicholds (MN), CEO at Newcells to find out how clients are using AI, how it is impacting Newcells’ services and how it might accelerate drug discovery in the coming years.
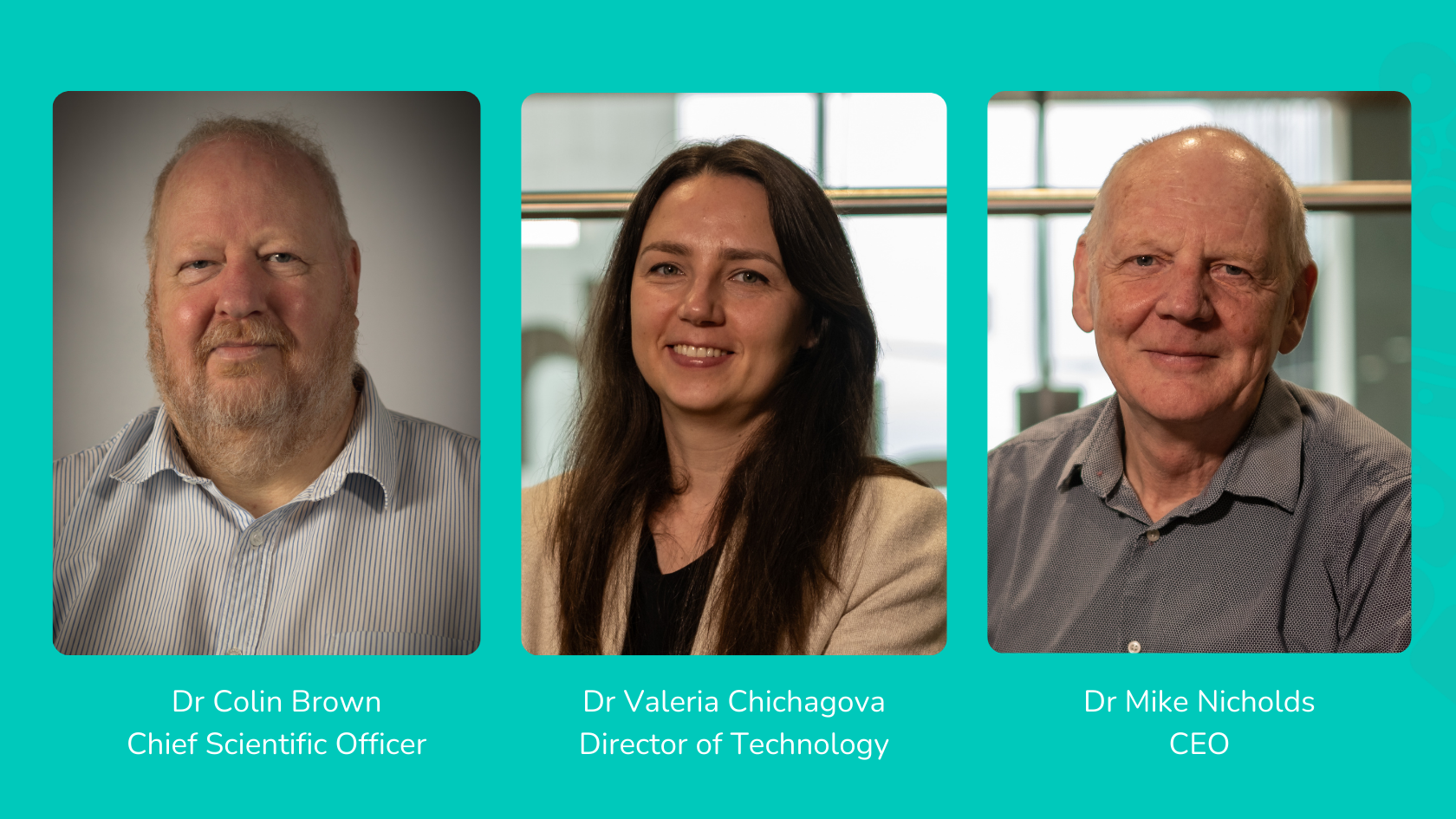
Colin Brown: “The role of AI in the discovery process will evolve over the next few years as there are many areas in which we can already begin to use it. Essentially, it is just using computing power. It is a mixture of bioinformatics and machine learning. So how do we use it? In the very early stages, you are looking at trying to design and pick out drugs. So essentially it is a better way of doing in silico selection because you are using information. For example, you can take a whole dataset of in silico 3D structures. Then you can look at very similar drugs analysing data sets from in the in vitro models and in vivo animal models. Then you can look at what has happened in the clinic with the similar types of drugs and then use that mega dataset to come up with information that allows you to really optimise drug design”.
Mike Nicholds: “I think over the next decade, AI will have an increasing impact on the drug discovery industry. At the moment, it is in its early stages with a lot of investment going in, particularly looking at areas where I think the impact can be quite large in the short term; for example, looking at processes that can be automated within the drug discovery pipeline. But also more excitingly, I guess there are several startups which are engaged with large pharma, looking at implementing AI in the fundamentals of drug discovery, which would be designing new molecules from scratch, using large data sets and models of proteins and targets. It is really quite innovative and quite exciting, because the output could be new drugs, which are new modalities, and potentially targeting previously undruggable targets, which would be really impactful for the industry”.
Valeria Chichagova: “ For me, it has already started. The current trends that we are already seeing in the field and working with our customers, is that there is a lot more focus on using AI across all stages of drug discovery. Starting from very early stages where identification and validation is done using huge data sets already available from various transcriptomics and proteomics analyses to try and understand which targets are more amenable in the context of various diseases that the companies are targeting. The next stage is drug design and optimization. This is where there might be a more integrative approach of using AI with some other novel technologies, such as quantum computing to get for example a very detailed analysis of the chemical structure of a drug. So, using the knowledge base that is already there and combining the two together to really look at the intricacies of drug design and make it much more specific than it currently is. Another stage is reached once the data based on the selected drug is obtained (in vitro or in vivo). How do you analyse this data? There is a lot more AI involvement to approach data analysis. So, whether it is high content imaging or other types of huge data sets, they now can be driven by AI in terms of analysis.
Then we go further down the line in the drug discovery process with clinical trials. How do we choose patient populations? There could be biomarker driven approaches to identifying the correct patient population which is more likely to respond to the drug that is being tested. These are the areas where AI can come into play on the patient stratification side. And then the next stage towards the final points of drug discovery process and late stages clinical trials, is again getting the data out of clinical trials. How do we analyse the data, especially at the later stages when the patient population is much higher?”
Have your clients used AI for their drug discovery programmes?
Valeria Chichagova: “Yes, some of our customers have so-called ‘virtual labs’. They don’t actually have any lab space or do any wet lab work themselves, but they do everything completely in silico. They look for targets and perform in silicon modelling. But then they need to validate their findings on different models. So, they come to us to do the validation work using our complex in vitro models. We do see that more and more in drug discovery, specifically for target identification and target validation. Also on the data analysis side, we have customers that have a large capability in obtaining high content data and high content imaging and I do know that they use AI in their analysis as well.”
Mike Nicholds: “We work with a range of clients, from large pharma to biotechs, and we know that some of them have already invested in AI. Particularly the large pharma companies are already investing both internally and in partnerships. So we anticipate that biotech companies also start to move down that track. We have worked with companies on AI projects in the areas of imaging, an area where in the short term we will have our own offering using AI in that space.”
Colin Brown: “It is very clear that the top pharma companies like Pfizer, AZ, GSK, have large active research programs using this. The problem for smaller pharma companies is actually having the computing power and also the knowledge to be able to use AI. So, you do need more specialised people such as bioinformaticians and bioanalysts. Large companies are certainly well down the route. They are driven in part by the fact that the FDA is now talking about how they can incorporate AI and how they are going to accept AI driven data.”
How has it impacted your services?
Colin Brown: “At the moment, we are screening compounds without actively using AI. How we could use AI, is to generate very large datasets. Something we would like to do is to use artificial intelligence and machine learning to analyse these large data sets that we produce in more detail to find trends that we are not currently seeing. Currently, it is in its infancy in our company, and I think we will move towards it as time progresses.”
Mike Nicholds: “At the moment, we see AI as a coming technology which can do a number of things. One is to improve productivity within the company. For example, in our imaging platform, we have invested recently in equipment which uses high end AI technology to help in the interpretation and quantification of imaging produced from our organoids. And also we are looking at ways to improve the automation of our data analysis to obtain more and better data. Obviously, the key for Newcells is to be able to take the data that we produce from our complex in vitro models, interpret it and give some really meaningful data back to the customers about what their molecule is doing in that particular tissue or organ.”
Valeria Chichagova: “One of the key offerings is to supply a larger amount of data, deliver it quicker and with higher predictive value, because you can train the system on our models. So, the more projects that we do, the more accurate the data becomes. I think we will deepen the offering that we already have, but also make it potentially larger in terms of the amount of data analysed and faster in terms of how quickly it is delivered. What this means for the customers, it de-risks their decision making. So, with the data quality being higher, they are in a better position to make the decision of taking a drug forward and how quickly to do it. We are already working with customers that are certainly using less animals in their drug discovery process through their trust in some of our models. If we can improve that even further with the data quality being higher based on integration of new technologies such as AI, it takes away even more of the risks that they are facing currently.
It could also impact timelines. We know that our customers are under lots of pressure, especially when they get closer to IND submission. We can turn things around quicker, so that they can make their decisions on time and trust that those decisions are going to be based on high quality data. Then the whole process will go smoother, and they will progress through the drug discovery pipeline quicker.”
Do you think it has accelerated their drug discovery?
Mike Nicholds: “I think it has. There are a number of examples where AI has already impacted the pharmaceutical industry even for drugs in the public domain. We have seen it from some examples with our customers who are clearly using the technology in areas of drug discovery. In the broader sense, AI is also impacting clinical trial work where you can use the data or the AI engines to improve your data analysis. In the preclinical space too we see examples of customers using the technology to automate some processes to help them analyse the large datasets they have access to.”
Valeria Chichagova: “Certainly yes, for some of them. We see a lot more AI currently and the adoption of AI across the industry will increase over time. For customers that solely focus on AI, is there technology in their innovation strategy? Yes, absolutely. So that certainly accelerated how quickly they can find the right targets or the right design of the drugs, and we will probablyl see more of that in the future.
Colin Brown: “It’s a relatively new process and given the drug discovery pipeline is typically a decade, it takes a long time to get from start to end. I am sure AI has accelerated drug discovery, particularly in the early years predicting 3D molecular structures. Also ,perhaps later in drug manufacturing as well. People are talking about how you can use AI to optimise the process of making a drug. So that is something very important as well. So, I think companies are beginning to use these things. Have we ever had a drug that is AI driven from the start to the end.? I’m not sure.”
How is Newcells planning to use AI in the coming years?
Mike Nicholds In the coming years, Newcells is planning to invest more in AI. I think for us, as we look forward over the next five years, our strategic planning really is focused initially on improving the ways that we can add value to our customers by better interpretation of the data sets. That includes having large data sets that we have accumulated over the years, and larger data sets to help us predict how the drug is going to interact with humans in clinical trials, which is clearly, from their point of view, the most important bits of data that they require from us.
Valeria Chichagova : “So our approach to AI use is more on the data analysis side initially. We have recently invested in an extensive imaging suite, which includes high content confocal microscopes. So that allows generation of huge amounts of data, and the data analysis comes with AI components. So we can train it on our models to then get more accurate data without building larger data sets. So for us, currently the use of AI is based on imaging, but I can see how we might potentially use it for other applications in the future as well.
Colin Brown: “Newcells generates a large volume of data in its assays. You are covering many variables. At the moment, we tend not to look at the linkage between variables at a deep level, and I think AI would allow us to do that, which may allow us to design our screening experiments to be more efficient and to give better results.”
Share on social media:
Don't miss out on our latest innovations: follow us on Linkedin